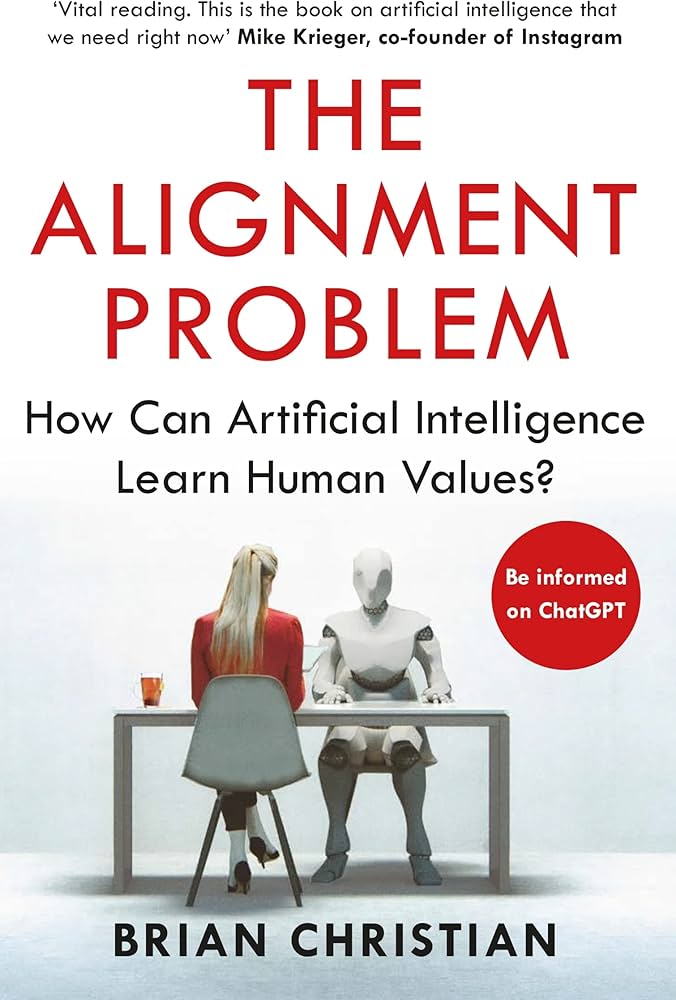
The Alignment Problem by Brian Christian book cover
AI at large
On how to build a middleground between machine learning and human values
This is a book about machine learning and human values: about systems that learn from data without being explicitly programmed, and about how exactly—and what exactly—we are trying to teach them.
From the first attempt at correlating human neurons to logical reasonning to extensive examples on how unassisted AI producing unfair outputs that jeopardize the ideal of justice, this book by Brian Christian explores what it takes to trust machine learning with decisions that (truly) affect humans. What is called the alignment problem is the mismatch between the output of an artifial intelligence and the true intention of its operator.
A few questions this book tries to give an answer to
- How can one align human values with the imprevisible output of an AI system?
- The bias on race, gender is hard to fix as AI is mostly based on what happened, not on what the society aims as an ideal of social justice, how to remedy that?
Who should read this book?
Anyone interested in the effects of a machine learning system on a human group or humans altogether. It's a must read for all AI enthusiasts and operators really.
Takeaways
- The historical examples are well expanded and illustrative, albeit prolixe
Bits
“This is a book about machine learning and human values: about systems that learn from data without being explicitly programmed, and about how exactly—and what exactly—we are trying to teach them.” Page 9
“The field of machine learning comprises three major areas: In unsupervised learning, a machine is simply given a heap of data and—as with the word2vec system—told to make sense of it, to find patterns, regularities, useful ways of condensing or representing or visualizing it. In supervised learning, the system is given a series of categorized or labeled examples—like parolees who went on to be rearrested and others who did not—and told to make predictions about new examples it hasn’t seen yet, or for which the ground truth is not yet known. And in reinforcement learning, the system is placed into an environment with rewards and punishments—like the boat-racing track with power-ups and hazards—and told to figure out the best way to minimize the punishments and maximize the rewards.”
“As machine-learning systems grow not just increasingly pervasive but increasingly powerful, we will find ourselves more and more often in the position of the “sorcerer’s apprentice”: we conjure a force, autonomous but totally compliant, give it a set of instructions, then scramble like mad to stop it once we realize our instructions are imprecise or incomplete—lest we get, in some clever, horrible way, precisely what we asked for. How to prevent such a catastrophic divergence—how to ensure that these models capture our norms and values, understand what we mean or intend, and, above all, do what we want—has emerged as one of the most central and most urgent scientific questions in the field of computer science. It has a name: the alignment problem.”